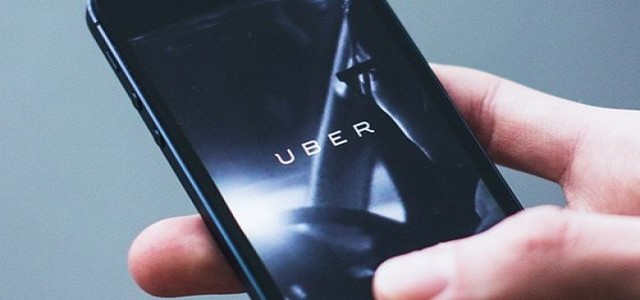
Uber, an American ride-hailing company, has recently published details of its VerCD technology, which is deployed for prototyping self-driving cars. The set of technologies is expected to help engineers, under its ATG (Advanced Technologies Group), validate, test, and deploy AI models to autonomous cars. However, the company is yet to publicly issue details regarding the architecture of the platform.
Uber’s driverless efforts suffered a serious setback after the Tempe, Arizona incident, when an autonomous car operated by the company caused a pedestrian fatality in 2018. Since then, the company has been focusing on advancing technology to upgrade the safety of self-driving cars.
VerCD is a set of microservices and tools developed for prototyping autonomous vehicles. It can track dependencies among various AI models, data sets, and codebases that are in the development phase. According to the ride-hailing company, VerCD is the most crucial component to maintain workflows, right from the data set extraction to the model serving stages. It also enables the interaction of the existing systems with the full, end-to-end ML workflow within ATG.
Through VerCD’s data set building workflow, fresh data set builds’ frequency has been increased by a factor of over 10, which in turn, increases the iteration speed of machine learning engineers. The company also has provided onboard daily as well as weekly training jobs to detect flagship object and develop path detection models.
The recent Orchestrator Service of VerCD can use data primitives to build autonomous vehicles’ runtime. Also, the service will interact with a code repository while replicating data sets to & from the cloud and between datacenters, as well as creating deep learning libraries of images.
Uber has noted that the smooth transition between production and experimental model will enforce more constraints on the model training to ensure traceability and reproducibility. VerCD caters to this system by supporting a validation step for this transition. Depending on the system performance, the tool will designate a model as failed, aborted, or successful, offering ML engineers the opportunity to rebuild parameter sets in case of aborted or failed models.
Source credit:
https://venturebeat.com/2020/03/04/uber-details-vercd-the-ai-tech-powering-its-self-driving-cars/